Understanding bias in recruitment AI
What is bias and why is it a problem?
The process of recruitment strives to find the perfect candidate for an employment position. Ideally the candidate should fulfill all required criteria to be able to execute the job as well as possible.
However, in reality human judgment tends to be less objective. In practice factors that are not necessary to execute the job satisfactorily will play a role in the selection process. For example, recruiters may take ethnicity, gender, familiar education institutions or companies into account when making such a decision, often without them even realizing it. The recruiter is in most cases not even aware of this effect. This is called ‘unconscious bias’.
Numerous studies have confirmed that in HR, unconscious bias is a significant factor in causing unfair distribution of opportunities and decreasing diversity on the labour market.
At Textkernel, we are dedicated to championing responsible AI in recruitment, placing a premium on ethical practices and inclusivity to pave the way for a brighter, more equitable future.
MITIGATING BIAS AND ENSURING RESPONSIBLE AI
Using AI can be a double-edged sword. It can cause harm when used carelessly, but it can equally promote fairness and reduce bias when employed responsibly – which is crucial to ensuring ethical outcomes.
REAL WORLD APPLICATIONS
Responsible use of AI in real world applications
Now that we’ve looked at how AI can be harmful when used carelessly, it’s time to look at how to use AI in a safe and ethical manner. This is called Responsible AI. In fact, when used responsibly AI can help reduce bias, instead of amplifying it.
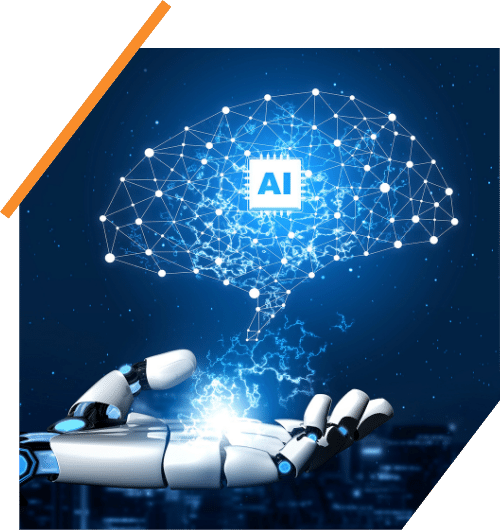
DESIGNING SYSTEMS AROUND USER AND AI BIAS
Mitigating bias in AI
Responsible AI in practice
The Textkernel solution
Document understanding
Enhance data extraction, standardization, and enrichment to reduce bias and improve document understanding.
Source & Match
Improve sourcing and matching precision with AI-driven search queries and enriched criteria which enhances search accuracy.
Responsible AI use
Ensure transparency and control in AI-driven processes to reduce bias and foster fair and ethical AI practices.
Reducing human unconscious bias
Textkernel aims to reduce disparities and foster fairness in critical decision-making processes.
Document understanding
The first step of any automated recruitment process is to understand the data. Our Parsing product is a perfect example of this. Understanding a document means to be able to extract the relevant information from a document and enrich it with domain specific knowledge. For example, when we parse a CV, the system reads what work experience the candidate has, but also which skills and degrees he or she possesses and so on (i.e. extraction ). On top of that, it can also standardize the job title and skills to existing taxonomies (i.e. normalization ), derive in which work field the candidate is working, or infer likely skills for that candidate, even though these things are not explicitly mentioned in the document (i.e. enrichment).
We can apply the same process of extraction and enrichment to a job posting, to give us the structured information for the job. In the case of job postings, this entails things like the required experience level, skills, and degree etcetera.
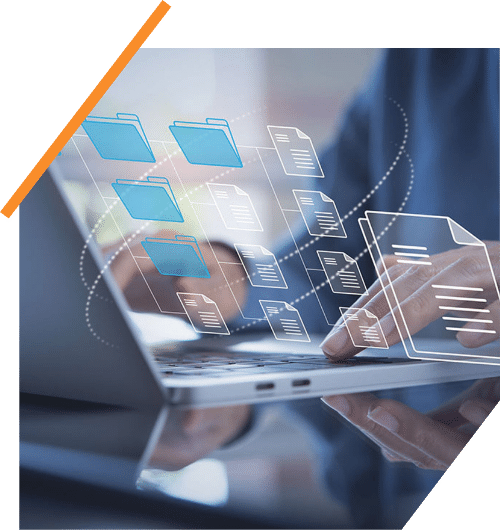
Searching and matching
This extracted and enriched knowledge is a very powerful tool for sourcing and matching. For example, understanding a document allows us to search only on professional skills instead of keyword matching on the entire document; or we can search on normalized job titles so we can find the candidate no matter how she/he expressed their job titles. This leads to a more accurate search. Another example is that we can search on inferred information (e.g. the experience level for a candidate, even though that experience level was not explicitly mentioned in their profile). Enrichment is useful not only for documents but also for search queries. For example, we can add synonyms or related terms to the search query.
Knowing all qualifications of candidates and all the requirements for job postings allows us to automate one more step: matching. To achieve this, we automatically generate a search query given an input document. Let’s say we want to match all suitable candidates for a given job, the search query will contain all required and desired criteria for that vacancy. Each criterion will have its own appropriate weight to optimize the quality of the result set of the query.
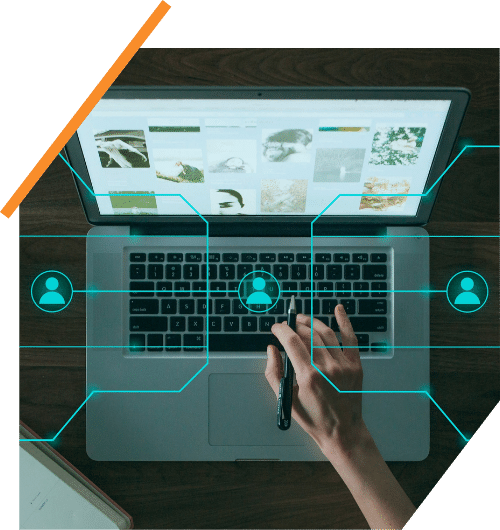
Responsible use of AI in Textkernel solution
Why does all this matter? Well, most importantly: AI doesn’t do matching for you. The matching is done in a term-based search engine. We employ powerful AI algorithms only for document understanding (to extract information and enrich documents and queries) but leave the matching part to more transparent and controllable algorithms. This way we give the recruiter full control over the matching, and benefit from our AI-driven world leading parsing capabilities.
However, even when employing transparent and controllable algorithms, bias may arise through properties of the language. For example, a simple term-based search on “waiter” will favor male candidates for that job, since the job title is male by definition. Enrichment of search and match queries helps reduce this type of bias. When recruiting for a waiter job, the query will be automatically enriched with the waitress job title to remove gender bias inherent to that job title. A similar bias reduction can be achieved by normalizing the job titles (as discussed before), normalizing skills and using it in queries: this ensures that no matter how the candidate expresses a skill or previous experience, the concept will still be matched.
To control any bias that could potentially arise in the AI-powered document understanding steps of the process, we enforce our R&D Fairness Checklist.
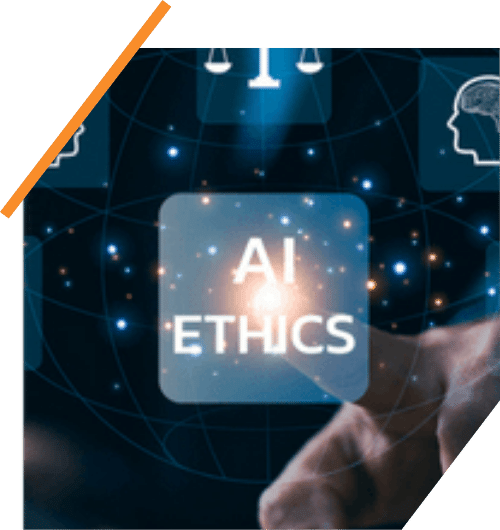
Reducing human unconscious bias
Having fully controllable and transparent matching has another benefit: by matching on objective criteria, we may actually mitigate any unconscious bias that a recruiter may have. This will improve equal opportunities and diversity in your HR processes.
Current research suggests that if used carefully, AI can help avoid discrimination, and even raise the bar for human decision-making.
Of course the user is unable to search on any discriminatory attributes when searching with Textkernel’s Source and Match, like gender or religion.
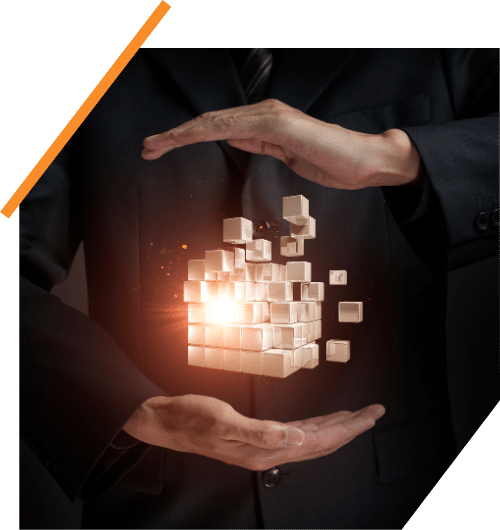
Guiding Textkernel’s ethical approach
Textkernel’s AI principles
At Textkernel, our approach to responsible AI is ingrained in our principles. We believe that AI should serve as a tool guided by humans, not an unsupervised decision maker. Transparency, diversity, and data security are all vitally important for us.